Unsupervised Learning of Shape-Motion Patterns for Objects in Urban Street Scenes
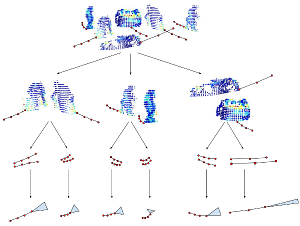
Tracking in urban street scenes is predominantly based on pretrained object-specific detectors and Kalman filter based tracking. More recently, methods have been proposed that track objects by modelling their shape, as well as ones that predict the motion of ob- jects using learned trajectory models. In this paper, we combine these ideas and propose shape-motion patterns (SMPs) that incorporate shape as well as motion to model a vari- ety of objects in an unsupervised way. By using shape, our method can learn trajectory models that distinguish object categories with distinct behaviour. We develop methods to classify objects into SMPs and to predict future motion. In experiments, we analyze our learned categorization and demonstrate superior performance of our motion predictions compared to a Kalman filter and a learned pure trajectory model. We also demonstrate how SMPs can indicate potentially harmful situations in traffic scenarios.
@inproceedings{klostermann2016_smps,
title = {Unsupervised Learning of Shape-Motion Patterns for Objects in Urban Street Scenes},
author = {Dirk Klostermann and Aljosa Osep and J\"org St\"uckler and Bastian Leibe},
booktitle = {Proc. of the British Machine Vision Conference (BMVC)},
year = {2016}, note = {to appear}
}