Multi-View Deep Learning for Consistent Semantic Mapping with RGB-D Cameras
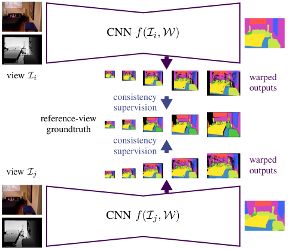
Visual scene understanding is an important capability that enables robots to purposefully act in their environment. In this paper, we propose a novel deep neural network approach to predict semantic segmentation from RGB-D sequences. The key innovation is to train our network to predict multi-view consistent semantics in a self-supervised way. At test time, its semantics predictions can be fused more consistently in semantic keyframe maps than predictions of a network trained on individual views. We base our network architecture on a recent single-view deep learning approach to RGB and depth fusion for semantic object-class segmentation and enhance it with multi-scale loss minimization. We obtain the camera trajectory using RGB-D SLAM and warp the predictions of RGB-D images into ground-truth annotated frames in order to enforce multi-view consistency during training. At test time, predictions from multiple views are fused into keyframes. We propose and analyze several methods for enforcing multi-view consistency during training and testing. We evaluate the benefit of multi-view consistency training and demonstrate that pooling of deep features and fusion over multiple views outperforms single-view baselines on the NYUDv2 benchmark for semantic segmentation. Our end-to-end trained network achieves state-of-the-art performance on the NYUDv2 dataset in single-view segmentation as well as multi-view semantic fusion.
@string{iros="International Conference on Intelligent Robots and Systems (IROS)"}
@InProceedings{lingni17iros,
author = "Lingni Ma and J\"org St\"uckler and Christian Kerl and Daniel Cremers",
title = "Multi-View Deep Learning for Consistent Semantic Mapping with RGB-D Cameras",
booktitle = "IEEE/RSJ Int. Conf. on Intelligent Robots and Systems (IROS)",
year = "2017",
}