Publications
Fine-Tuning Image-Conditional Diffusion Models is Easier than You Think

Recent work showed that large diffusion models can be reused as highly precise monocular depth estimators by casting depth estimation as an image-conditional image generation task. While the proposed model achieved state-of-the-art results, high computational demands due to multi-step inference limited its use in many scenarios. In this paper, we show that the perceived inefficiency was caused by a flaw in the inference pipeline that has so far gone unnoticed. The fixed model performs comparably to the best previously reported configuration while being more than 200x faster. To optimize for downstream task performance, we perform end-to-end fine-tuning on top of the single-step model with task-specific losses and get a deterministic model that outperforms all other diffusion-based depth and normal estimation models on common zero-shot benchmarks. We surprisingly find that this fine-tuning protocol also works directly on Stable Diffusion and achieves comparable performance to current state-of-the-art diffusion-based depth and normal estimation models, calling into question some of the conclusions drawn from prior works.
@article{martingarcia2024diffusione2eft,
title = {Fine-Tuning Image-Conditional Diffusion Models is Easier than You Think},
author = {Martin Garcia, Gonzalo and Abou Zeid, Karim and Schmidt, Christian and de Geus, Daan and Hermans, Alexander and Leibe, Bastian},
journal = {arXiv preprint arXiv:2409.11355},
year = {2024}
}
Interactive4D: Interactive 4D LiDAR Segmentation

Interactive segmentation has an important role in facilitating the annotation process of future LiDAR datasets. Existing approaches sequentially segment individual objects at each LiDAR scan, repeating the process throughout the entire sequence, which is redundant and ineffective. In this work, we propose interactive 4D segmentation, a new paradigm that allows segmenting multiple objects on multiple LiDAR scans simultaneously, and Interactive4D, the first interactive 4D segmentation model that segments multiple objects on superimposed consecutive LiDAR scans in a single iteration by utilizing the sequential nature of LiDAR data. While performing interactive segmentation, our model leverages the entire space-time volume, leading to more efficient segmentation. Operating on the 4D volume, it directly provides consistent instance IDs over time and also simplifies tracking annotations. Moreover, we show that click simulations are crucial for successful model training on LiDAR point clouds. To this end, we design a click simulation strategy that is better suited for the characteristics of LiDAR data. To demonstrate its accuracy and effectiveness, we evaluate Interactive4D on multiple LiDAR datasets, where Interactive4D achieves a new state-of-the-art by a large margin.
@article{fradlin2024interactive4d,
title = {{Interactive4D: Interactive 4D LiDAR Segmentation}},
author = {Fradlin, Ilya and Zulfikar, Idil Esen and Yilmaz, Kadir and Kontogianni, Thodora and Leibe, Bastian},
journal = {arXiv preprint arXiv:2410.08206},
year = {2024}
}
Look Gauss, No Pose: Novel View Synthesis using Gaussian Splatting without Accurate Pose Initialization
3D Gaussian Splatting has recently emerged as a powerful tool for fast and accurate novel-view synthesis from a set of posed input images. However, like most novel-view synthesis approaches, it relies on accurate camera pose information, limiting its applicability in real-world scenarios where acquiring accurate camera poses can be challenging or even impossible. We propose an extension to the 3D Gaussian Splatting framework by optimizing the extrinsic camera parameters with respect to photometric residuals. We derive the analytical gradients and integrate their computation with the existing high-performance CUDA implementation. This enables downstream tasks such as 6-DoF camera pose estimation as well as joint reconstruction and camera refinement. In particular, we achieve rapid convergence and high accuracy for pose estimation on real-world scenes. Our method enables fast reconstruction of 3D scenes without requiring accurate pose information by jointly optimizing geometry and camera poses, while achieving state-of-the-art results in novel-view synthesis. Our approach is considerably faster to optimize than most com- peting methods, and several times faster in rendering. We show results on real-world scenes and complex trajectories through simulated environments, achieving state-of-the-art results on LLFF while reducing runtime by two to four times compared to the most efficient competing method. Source code will be available at https://github.com/Schmiddo/noposegs.
Point-VOS: Pointing Up Video Object Segmentation
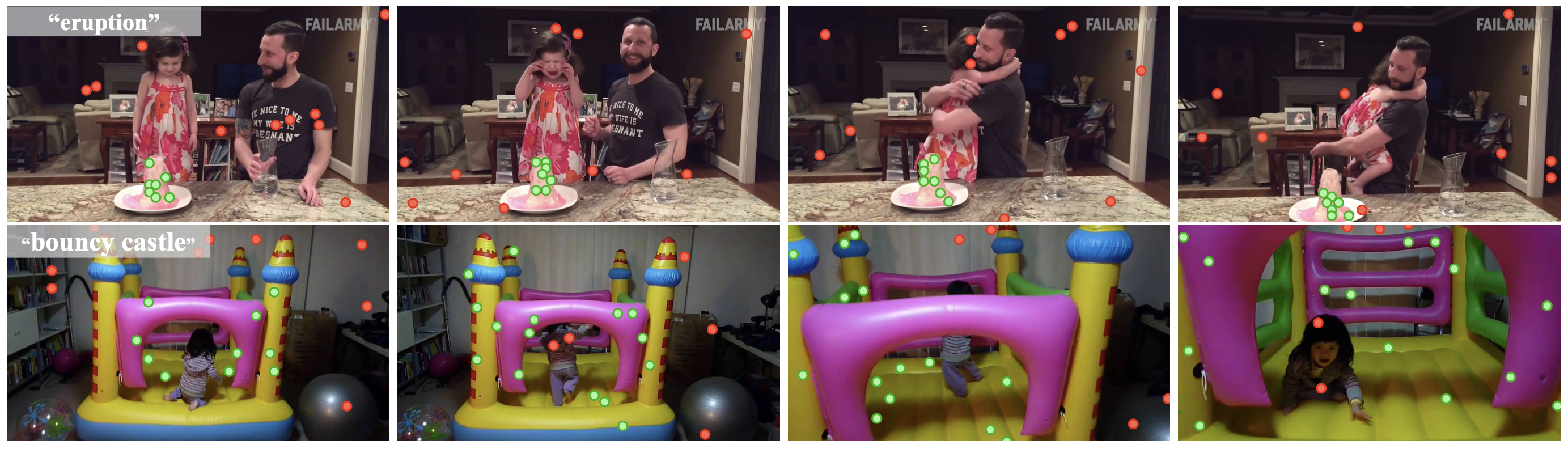
Current state-of-the-art Video Object Segmentation (VOS) methods rely on dense per-object mask annotations both during training and testing. This requires time-consuming and costly video annotation mechanisms. We propose a novel Point-VOS task with a spatio-temporally sparse point-wise annotation scheme that substantially reduces the annotation effort. We apply our annotation scheme to two large-scale video datasets with text descriptions and annotate over 19M points across 133K objects in 32K videos. Based on our annotations, we propose a new Point-VOS benchmark, and a corresponding point-based training mechanism, which we use to establish strong baseline results. We show that existing VOS methods can easily be adapted to leverage our point annotations during training, and can achieve results close to the fully-supervised performance when trained on pseudo-masks generated from these points. In addition, we show that our data can be used to improve models that connect vision and language, by evaluating it on the Video Narrative Grounding (VNG) task. We will make our code and annotations available at https://pointvos.github.io.
ControlRoom3D: Room Generation using Semantic Proxies
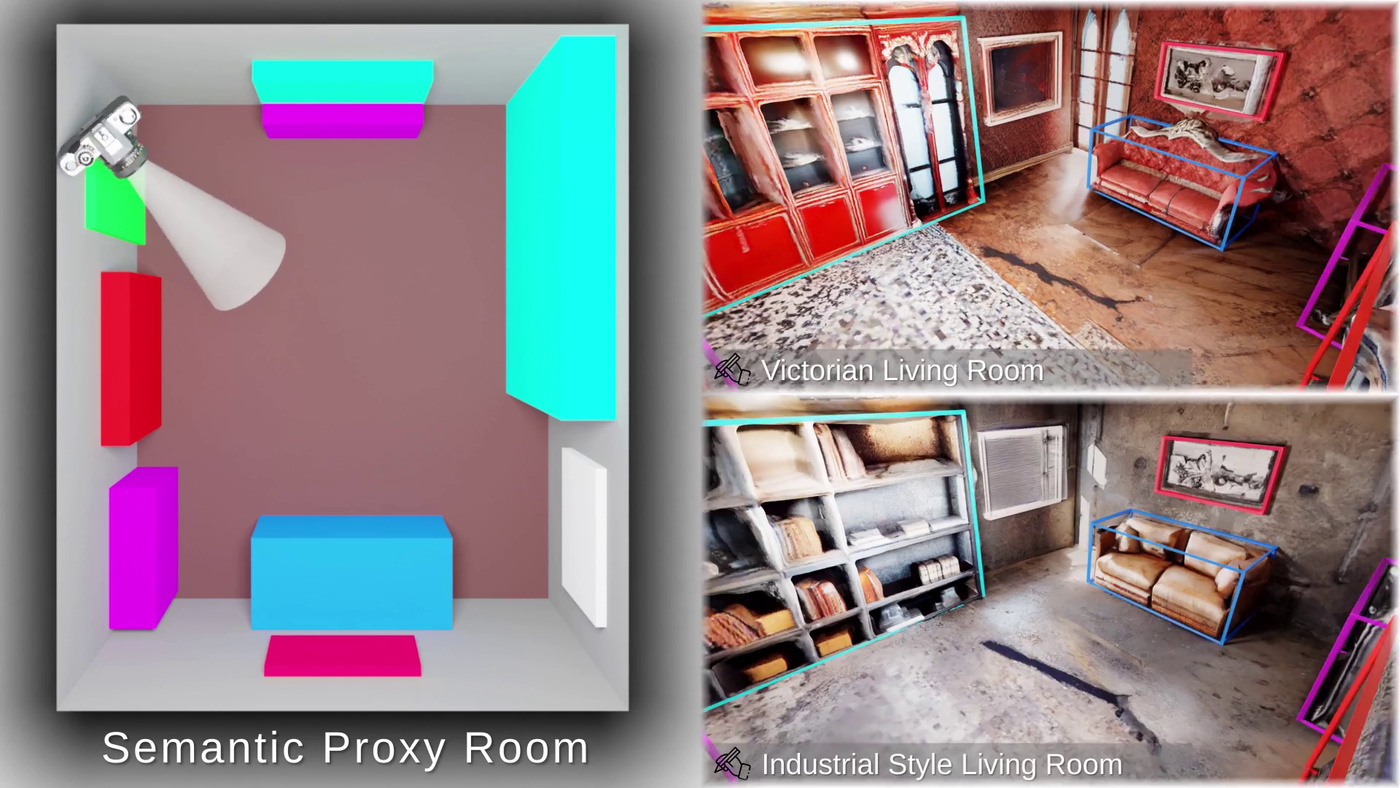
Manually creating 3D environments for AR/VR applications is a complex process requiring expert knowledge in 3D modeling software. Pioneering works facilitate this process by generating room meshes conditioned on textual style descriptions. Yet, many of these automatically generated 3D meshes do not adhere to typical room layouts, compromising their plausibility, e.g., by placing several beds in one bedroom. To address these challenges, we present ControlRoom3D, a novel method to generate high-quality room meshes. Central to our approach is a user-defined 3D semantic proxy room that outlines a rough room layout based on semantic bounding boxes and a textual description of the overall room style. Our key insight is that when rendered to 2D, this 3D representation provides valuable geometric and semantic information to control powerful 2D models to generate 3D consistent textures and geometry that aligns well with the proxy room. Backed up by an extensive study including quantitative metrics and qualitative user evaluations, our method generates diverse and globally plausible 3D room meshes, thus empowering users to design 3D rooms effortlessly without specialized knowledge.
@inproceedings{schult23controlroom3d,
author = {Schult, Jonas and Tsai, Sam and H\"ollein, Lukas and Wu, Bichen and Wang, Jialiang and Ma, Chih-Yao and Li, Kunpeng and Wang, Xiaofang and Wimbauer, Felix and He, Zijian and Zhang, Peizhao and Leibe, Bastian and Vajda, Peter and Hou, Ji},
title = {ControlRoom3D: Room Generation using Semantic Proxy Rooms},
booktitle = {IEEE Conference on Computer Vision and Pattern Recognition (CVPR)},
year = {2024},
}
Mask4Former: Mask Transformer for 4D Panoptic Segmentation
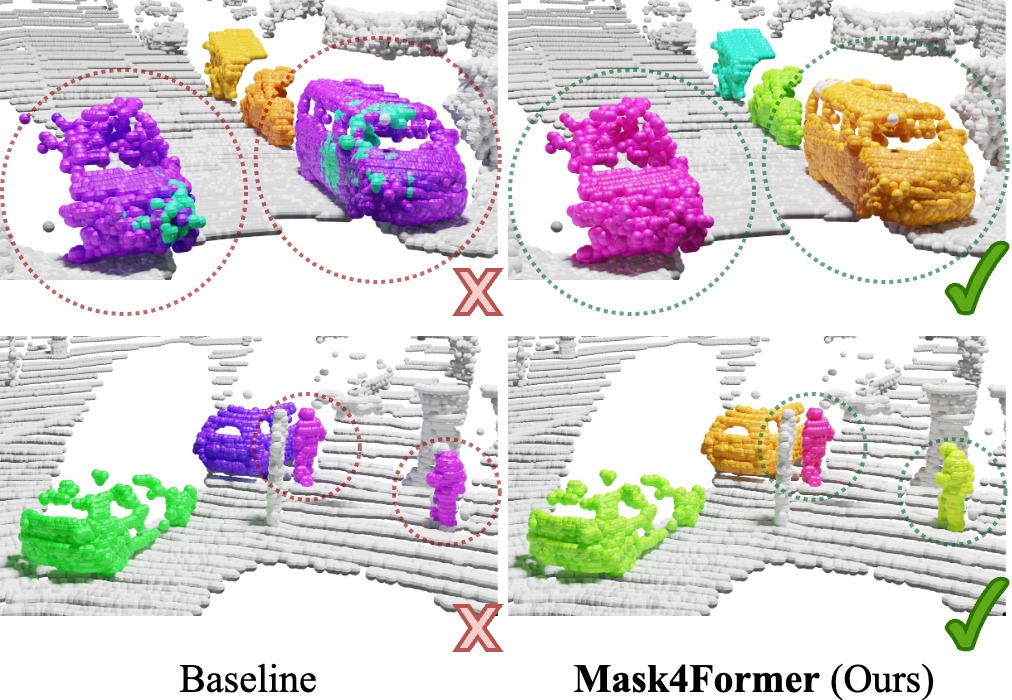
Accurately perceiving and tracking instances over time is essential for the decision-making processes of autonomous agents interacting safely in dynamic environments. With this intention, we propose Mask4Former for the challenging task of 4D panoptic segmentation of LiDAR point clouds.
Mask4Former is the first transformer-based approach unifying semantic instance segmentation and tracking of sparse and irregular sequences of 3D point clouds into a single joint model. Our model directly predicts semantic instances and their temporal associations without relying on hand-crafted non-learned association strategies such as probabilistic clustering or voting-based center prediction. Instead, Mask4Former introduces spatio-temporal instance queries that encode the semantic and geometric properties of each semantic tracklet in the sequence.
In an in-depth study, we find that promoting spatially compact instance predictions is critical as spatio-temporal instance queries tend to merge multiple semantically similar instances, even if they are spatially distant. To this end, we regress 6-DOF bounding box parameters from spatio-temporal instance queries, which are used as an auxiliary task to foster spatially compact predictions.
Mask4Former achieves a new state-of-the-art on the SemanticKITTI test set with a score of 68.4 LSTQ.
@inproceedings{yilmaz24mask4former,
title = {{Mask4Former: Mask Transformer for 4D Panoptic Segmentation}},
author = {Yilmaz, Kadir and Schult, Jonas and Nekrasov, Alexey and Leibe, Bastian},
booktitle = {International Conference on Robotics and Automation (ICRA)},
year = {2024}
}
AGILE3D: Attention Guided Interactive Multi-object 3D Segmentation
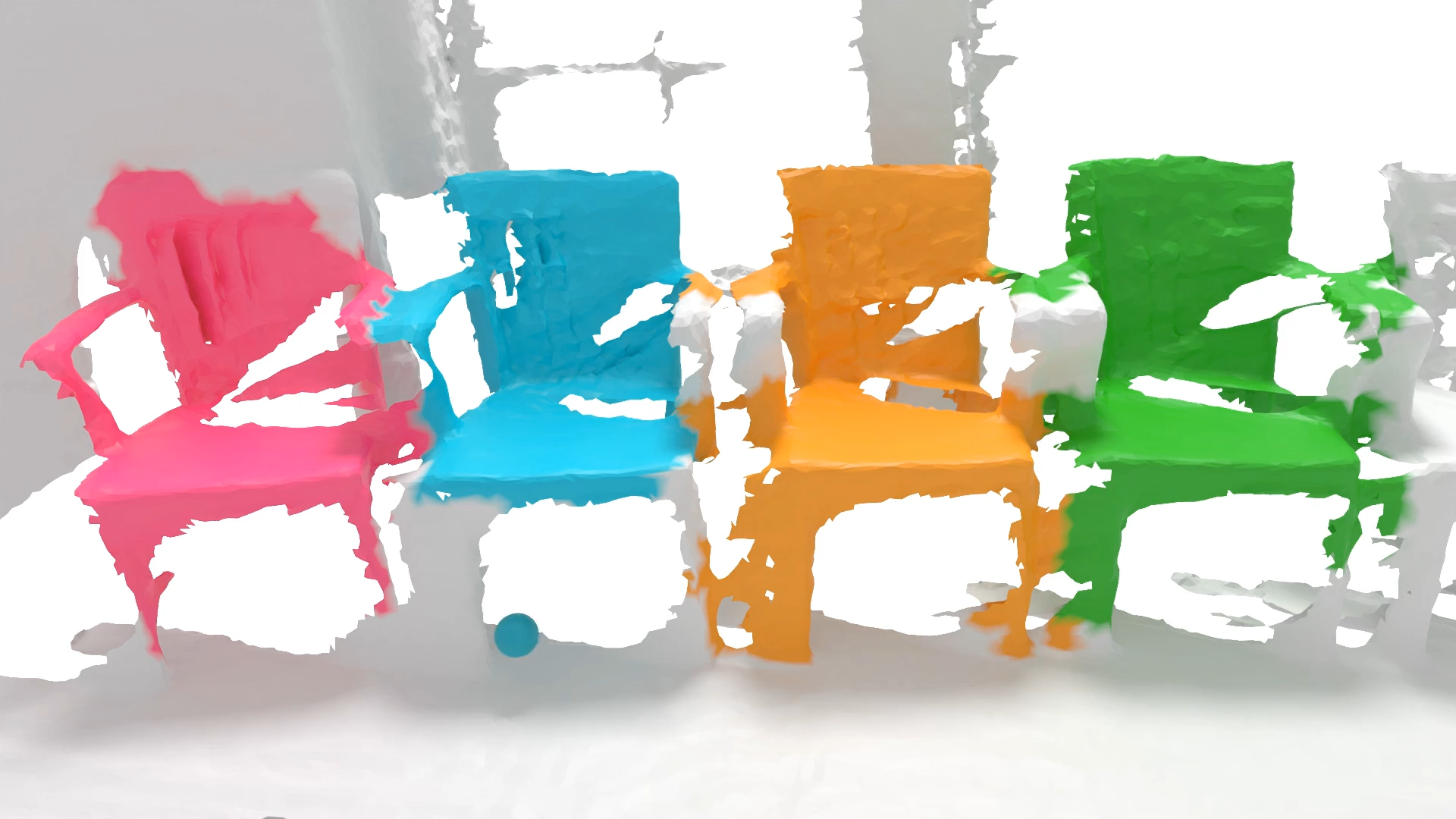
During interactive segmentation, a model and a user work together to delineate objects of interest in a 3D point cloud. In an iterative process, the model assigns each data point to an object (or the background), while the user corrects errors in the resulting segmentation and feeds them back into the model. The current best practice formulates the problem as binary classification and segments objects one at a time. The model expects the user to provide positive clicks to indicate regions wrongly assigned to the background and negative clicks on regions wrongly assigned to the object. Sequentially visiting objects is wasteful since it disregards synergies between objects: a positive click for a given object can, by definition, serve as a negative click for nearby objects. Moreover, a direct competition between adjacent objects can speed up the identification of their common boundary. We introduce AGILE3D, an efficient, attention-based model that (1) supports simultaneous segmentation of multiple 3D objects, (2) yields more accurate segmentation masks with fewer user clicks, and (3) offers faster inference. Our core idea is to encode user clicks as spatial-temporal queries and enable explicit interactions between click queries as well as between them and the 3D scene through a click attention module. Every time new clicks are added, we only need to run a lightweight decoder that produces updated segmentation masks. In experiments with four different 3D point cloud datasets, AGILE3D sets a new state-of-the-art. Moreover, we also verify its practicality in real-world setups with real user studies.
@inproceedings{yue2023agile3d,
title = {{AGILE3D: Attention Guided Interactive Multi-object 3D Segmentation}},
author = {Yue, Yuanwen and Mahadevan, Sabarinath and Schult, Jonas and Engelmann, Francis and Leibe, Bastian and Schindler, Konrad and Kontogianni, Theodora},
booktitle = {International Conference on Learning Representations (ICLR)},
year = {2024}
}
RGB-D Cube R-CNN: 3D Object Detection with Selective Modality Dropout

In this paper we create an RGB-D 3D object detector targeted at indoor robotics use cases where one modality may be unavailable due to a specific sensor setup or a sensor failure. We incorporate RGB and depth fusion into the recent Cube R-CNN framework with support for selective modality dropout. To train this model we augment the Omni3DIN dataset with depth information leading to a diverse dataset for 3D object detection in indoor scenes. In order to leverage strong pretrained networks we investigate the viability of Transformer-based backbones (Swin ViT) as an alternative to the currently popular CNN-based DLA backbone. We show that these Transformer-based image models work well based on our early-fusion approach and propose a modality dropout scheme to avoid the disregard of any modality during training facilitating selective modality dropout during inference. In extensive experiments our proposed RGB-D Cube R-CNN outperforms an RGB-only Cube R-CNN baseline by a significant margin on the task of indoor object detection. Additionally we observe a slight performance boost from the RGB-D training when inferring on only one modality which could for example be valuable in robotics applications with a reduced or unreliable sensor set.
@InProceedings{RGB_D_Cube_RCNN_2024_CVPRW,
author = {Piekenbrinck, Jens and Hermans, Alexander and Vaskevicius, Narunas and Linder, Timm and Leibe, Bastian},
title = {{RGB-D Cube R-CNN: 3D Object Detection with Selective Modality Dropout}},
booktitle = {Proceedings of the IEEE/CVF Conference on Computer Vision and Pattern Recognition (CVPR) Workshops},
year = {2024},
}
OoDIS: Anomaly Instance Segmentation Benchmark
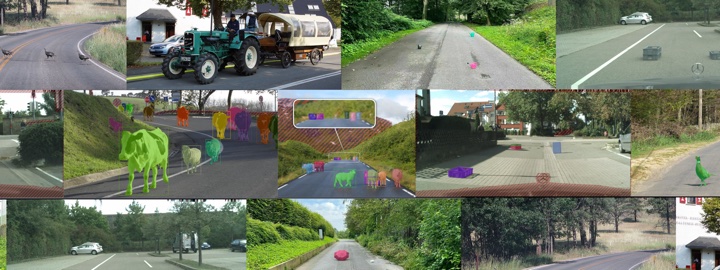
Autonomous vehicles require a precise understanding of their environment to navigate safely. Reliable identification of unknown objects, especially those that are absent during training, such as wild animals, is critical due to their potential to cause serious accidents. Significant progress in semantic segmentation of anomalies has been driven by the availability of out-of-distribution (OOD) benchmarks. However, a comprehensive understanding of scene dynamics requires the segmentation of individual objects, and thus the segmentation of instances is essential. Development in this area has been lagging, largely due to the lack of dedicated benchmarks. To address this gap, we have extended the most commonly used anomaly segmentation benchmarks to include the instance segmentation task. Our evaluation of anomaly instance segmentation methods shows that this challenge remains an unsolved problem. The benchmark website and the competition page can be found at: https://vision.rwth-aachen.de/oodis
@inproceedings{nekrasov2023ugains,
title = {{UGainS: Uncertainty Guided Anomaly Instance Segmentation}},
author = {Nekrasov, Alexey and Hermans, Alexander and Kuhnert, Lars and Leibe, Bastian},
booktitle = {GCPR},
year = {2023}
}
Previous Year (2023)