Mask4Former: Mask Transformer for 4D Panoptic Segmentation
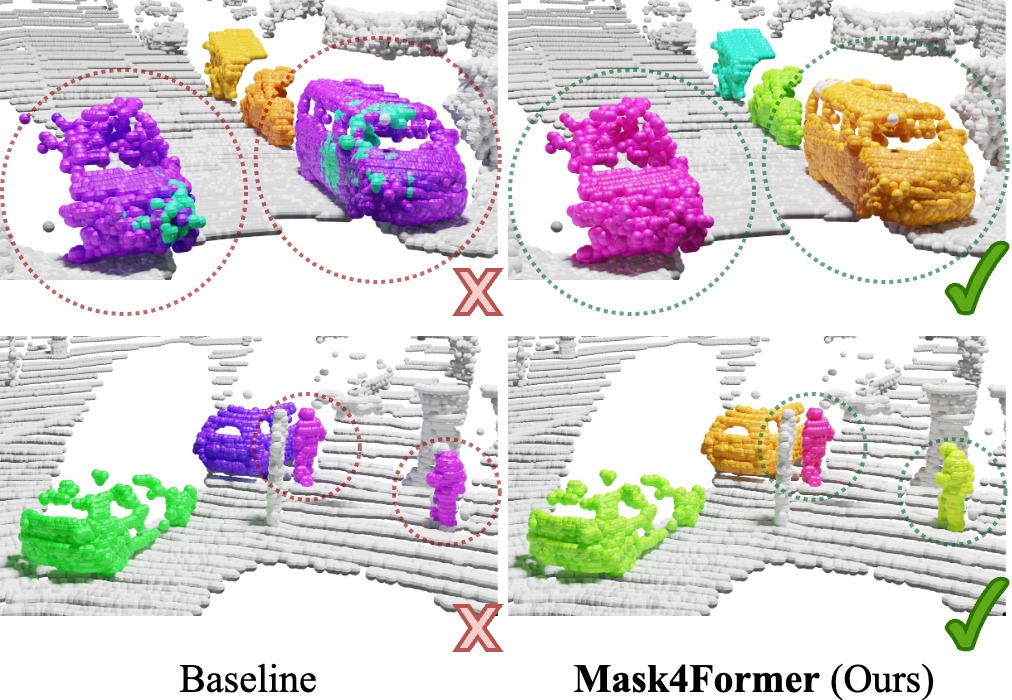
Accurately perceiving and tracking instances over time is essential for the decision-making processes of autonomous agents interacting safely in dynamic environments. With this intention, we propose Mask4Former for the challenging task of 4D panoptic segmentation of LiDAR point clouds.
Mask4Former is the first transformer-based approach unifying semantic instance segmentation and tracking of sparse and irregular sequences of 3D point clouds into a single joint model. Our model directly predicts semantic instances and their temporal associations without relying on hand-crafted non-learned association strategies such as probabilistic clustering or voting-based center prediction. Instead, Mask4Former introduces spatio-temporal instance queries that encode the semantic and geometric properties of each semantic tracklet in the sequence.
In an in-depth study, we find that promoting spatially compact instance predictions is critical as spatio-temporal instance queries tend to merge multiple semantically similar instances, even if they are spatially distant. To this end, we regress 6-DOF bounding box parameters from spatio-temporal instance queries, which are used as an auxiliary task to foster spatially compact predictions.
Mask4Former achieves a new state-of-the-art on the SemanticKITTI test set with a score of 68.4 LSTQ.
@inproceedings{yilmaz24mask4former,
title = {{Mask4Former: Mask Transformer for 4D Panoptic Segmentation}},
author = {Yilmaz, Kadir and Schult, Jonas and Nekrasov, Alexey and Leibe, Bastian},
booktitle = {International Conference on Robotics and Automation (ICRA)},
year = {2024}
}