Publications
MaskLab: Instance Segmentation by Refining Object Detection with Semantic and Direction Features
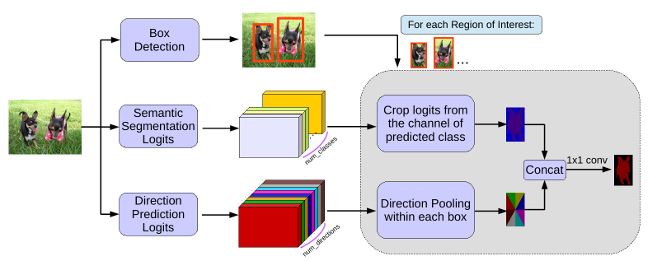
In this work, we tackle the problem of instance segmentation, the task of simultaneously solving object detection and semantic segmentation. Towards this goal, we present a model, called MaskLab, which produces three outputs: box detection, semantic segmentation, and direction prediction. Building on top of the Faster-RCNN object detector, the predicted boxes provide accurate localization of object instances. Within each region of interest, MaskLab performs foreground/background segmentation by combining semantic and direction prediction. Semantic segmentation assists the model in distinguishing between objects of different semantic classes including background, while the direction prediction, estimating each pixel's direction towards its corresponding center, allows separating instances of the same semantic class. Moreover, we explore the effect of incorporating recent successful methods from both segmentation and detection (i.e. atrous convolution and hypercolumn). Our proposed model is evaluated on the COCO instance segmentation benchmark and shows comparable performance with other state-of-art models.
@article{Chen18CVPR,
title = {{MaskLab: Instance Segmentation by Refining Object Detection with Semantic and Direction Features}},
author = {Chen, Liang-Chieh and Hermans, Alexander and Papandreou, George and Schroff, Florian and Wang, Peng and Adam, Hartwig},
journal = {{IEEE Conference on Computer Vision and Pattern Recognition (CVPR'18)}},,
year = {2018}
}
Deep Person Detection in 2D Range Data
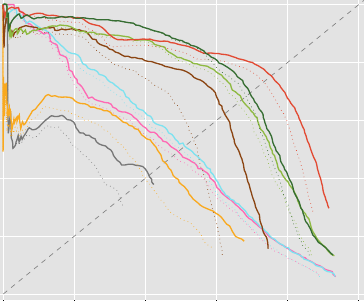
TL;DR: Extend the DROW dataset to persons, extend the method to include short temporal context, and extensively benchmark all available methods.
Detecting humans is a key skill for mobile robots and intelligent vehicles in a large variety of applications. While the problem is well studied for certain sensory modalities such as image data, few works exist that address this detection task using 2D range data. However, a widespread sensory setup for many mobile robots in service and domestic applications contains a horizontally mounted 2D laser scanner. Detecting people from 2D range data is challenging due to the speed and dynamics of human leg motion and the high levels of occlusion and self-occlusion particularly in crowds of people. While previous approaches mostly relied on handcrafted features, we recently developed the deep learning based wheelchair and walker detector DROW. In this paper, we show the generalization to people, including small modifications that significantly boost DROW's performance. Additionally, by providing a small, fully online temporal window in our network, we further boost our score. We extend the DROW dataset with person annotations, making this the largest dataset of person annotations in 2D range data, recorded during several days in a real-world environment with high diversity. Extensive experiments with three current baseline methods indicate it is a challenging dataset, on which our improved DROW detector beats the current state-of-the-art.
@article{Beyer2018RAL,
title = {{Deep Person Detection in 2D Range Data}},
author = {Beyer, Lucas and Hermans, Alexander and Linder, Timm and Arras, Kai Oliver and Leibe, Bastian},
journal = {IEEE Robotics and Automation Letters},
volume = {3},
number = {3},
pages = {2726--2733}
year = {2018}
}
Previous Year (2017)