Profile
![]() |
Dr. Francis Engelmann |
Students
Current
- Mats Steinweg (student worker)
- Nikolay Paleshnikov (student worker)
Past
-
Kushal Sharma Master Thesis
-
Mathias Pfeiffer (student worker)
-
Cathrin Elich Paper Master Thesis (DAGM Best Master Thesis Award)
-
Marvin Pförtner Bachelor Thesis
-
Anton Kasyanov Paper Master Thesis
-
Oleg Chernikov Master Thesis
Teaching
- Winter 2018: Computer Vision
- Winter 2017: Machine Learning
- Winter 2016: Introduction to Computer science
- Summer 2016: Computer Vision 2
- Winter 2014: Introduction to Computer science
Publications
AGILE3D: Attention Guided Interactive Multi-object 3D Segmentation
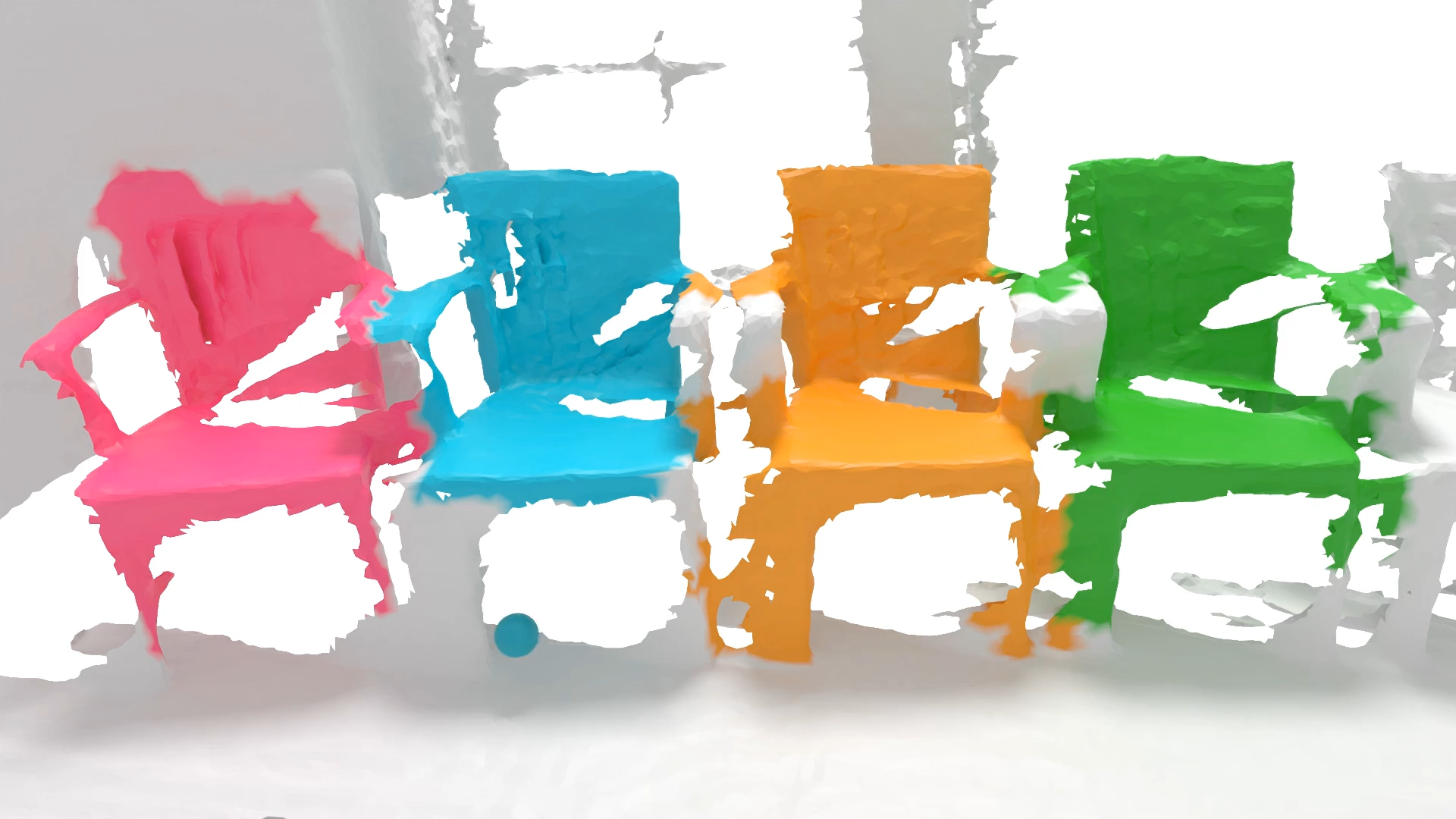
During interactive segmentation, a model and a user work together to delineate objects of interest in a 3D point cloud. In an iterative process, the model assigns each data point to an object (or the background), while the user corrects errors in the resulting segmentation and feeds them back into the model. The current best practice formulates the problem as binary classification and segments objects one at a time. The model expects the user to provide positive clicks to indicate regions wrongly assigned to the background and negative clicks on regions wrongly assigned to the object. Sequentially visiting objects is wasteful since it disregards synergies between objects: a positive click for a given object can, by definition, serve as a negative click for nearby objects. Moreover, a direct competition between adjacent objects can speed up the identification of their common boundary. We introduce AGILE3D, an efficient, attention-based model that (1) supports simultaneous segmentation of multiple 3D objects, (2) yields more accurate segmentation masks with fewer user clicks, and (3) offers faster inference. Our core idea is to encode user clicks as spatial-temporal queries and enable explicit interactions between click queries as well as between them and the 3D scene through a click attention module. Every time new clicks are added, we only need to run a lightweight decoder that produces updated segmentation masks. In experiments with four different 3D point cloud datasets, AGILE3D sets a new state-of-the-art. Moreover, we also verify its practicality in real-world setups with real user studies.
@inproceedings{yue2023agile3d,
title = {{AGILE3D: Attention Guided Interactive Multi-object 3D Segmentation}},
author = {Yue, Yuanwen and Mahadevan, Sabarinath and Schult, Jonas and Engelmann, Francis and Leibe, Bastian and Schindler, Konrad and Kontogianni, Theodora},
booktitle = {International Conference on Learning Representations (ICLR)},
year = {2024}
}
3D Segmentation of Humans in Point Clouds with Synthetic Data
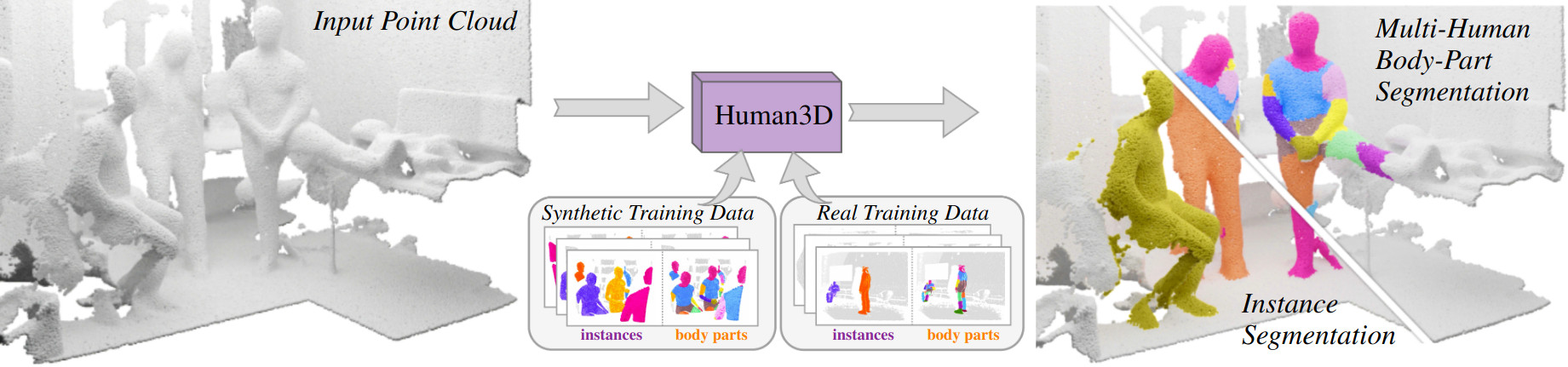
Segmenting humans in 3D indoor scenes has become increasingly important with the rise of human-centered robotics and AR/VR applications. In this direction, we explore the tasks of 3D human semantic-, instance- and multi-human body-part segmentation. Few works have attempted to directly segment humans in point clouds (or depth maps), which is largely due to the lack of training data on humans interacting with 3D scenes. We address this challenge and propose a framework for synthesizing virtual humans in realistic 3D scenes. Synthetic point cloud data is attractive since the domain gap between real and synthetic depth is small compared to images. Our analysis of different training schemes using a combination of synthetic and realistic data shows that synthetic data for pre-training improves performance in a wide variety of segmentation tasks and models. We further propose the first end-to-end model for 3D multi-human body-part segmentation, called Human3D, that performs all the above segmentation tasks in a unified manner. Remarkably, Human3D even outperforms previous task-specific state-of-the-art methods. Finally, we manually annotate humans in test scenes from EgoBody to compare the proposed training schemes and segmentation models.
@article{Takmaz23,
title = {{3D Segmentation of Humans in Point Clouds with Synthetic Data}},
author = {Takmaz, Ay\c{c}a and Schult, Jonas and Kaftan, Irem and Ak\c{c}ay, Mertcan
and Leibe, Bastian and Sumner, Robert and Engelmann, Francis and Tang, Siyu},
booktitle = {{International Conference on Computer Vision (ICCV)}},
year = {2023}
}
Mask3D for 3D Semantic Instance Segmentation
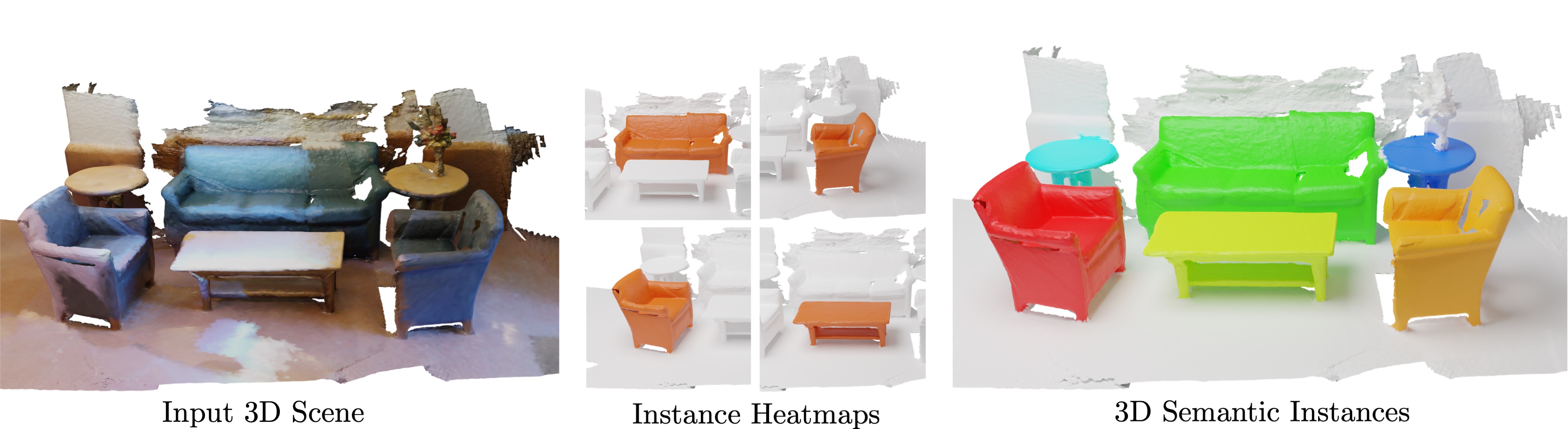
Modern 3D semantic instance segmentation approaches predominantly rely on specialized voting mechanisms followed by carefully designed geometric clustering techniques. Building on the successes of recent Transformer-based methods for object detection and image segmentation, we propose the first Transformer-based approach for 3D semantic instance segmentation. We show that we can leverage generic Transformer building blocks to directly predict instance masks from 3D point clouds. In our model called Mask3D each object instance is represented as an instance query. Using Transformer decoders, the instance queries are learned by iteratively attending to point cloud features at multiple scales. Combined with point features, the instance queries directly yield all instance masks in parallel. Mask3D has several advantages over current state-of-the-art approaches, since it neither relies on (1) voting schemes which require hand-selected geometric properties (such as centers) nor (2) geometric grouping mechanisms requiring manually-tuned hyper-parameters (e.g. radii) and (3) enables a loss that directly optimizes instance masks. Mask3D sets a new state-of-the-art on ScanNet test (+6.2 mAP), S3DIS 6-fold (+10.1 mAP), STPLS3D (+11.2 mAP) and ScanNet200 test (+12.4 mAP).
» Show BibTeX
@article{Schult23ICRA,
title = {{Mask3D for 3D Semantic Instance Segmentation}},
author = {Schult, Jonas and Engelmann, Francis and Hermans, Alexander and Litany, Or and Tang, Siyu and Leibe, Bastian},
booktitle = {{International Conference on Robotics and Automation (ICRA)}},
year = {2023}
}
4D-StOP: Panoptic Segmentation of 4D LiDAR using Spatio-temporal Object Proposal Generation and Aggregation
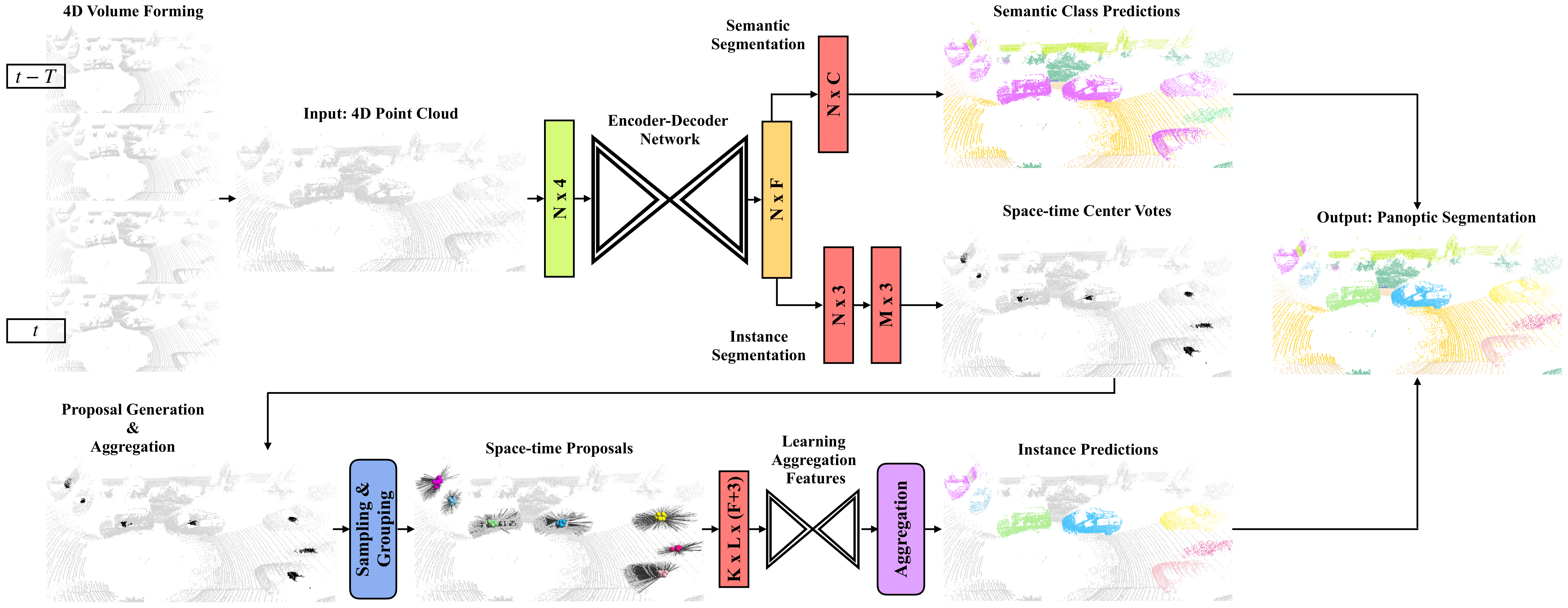
In this work, we present a new paradigm, called 4D-StOP, to tackle the task of 4D Panoptic LiDAR Segmentation. 4D-StOP first generates spatio-temporal proposals using voting-based center predictions, where each point in the 4D volume votes for a corresponding center. These tracklet proposals are further aggregated using learned geometric features. The tracklet aggregation method effectively generates a video-level 4D scene representation over the entire space-time volume. This is in contrast to existing end-to-end trainable state-of-the-art approaches which use spatio-temporal embeddings that are represented by Gaussian probability distributions. Our voting-based tracklet generation method followed by geometric feature-based aggregation generates significantly improved panoptic LiDAR segmentation quality when compared to modeling the entire 4D volume using Gaussian probability distributions. 4D-StOP achieves a new state-of-the-art when applied to the SemanticKITTI test dataset with a score of 63.9 LSTQ, which is a large (+7%) improvement compared to current best-performing end-to-end trainable methods. The code and pre-trained models are available at:https://github.com/LarsKreuzberg/4D-StOP
M2F3D: Mask2Former for 3D Instance Segmentation
In this work, we show that the top performing Mask2Former approach for image-based segmentation tasks works surprisingly well when adapted to the 3D scene understanding domain. Current 3D semantic instance segmentation methods rely largely on predicting centers followed by clustering approaches and little progress has been made in applying transformer-based approaches to this task. We show that with small modifications to the Mask2Former approach for 2D, we can create a 3D instance segmentation approach, without the need for highly 3D specific components or carefully hand-engineered hyperparameters. Initial experiments with our M2F3D model on the ScanNet benchmark are very promising and sets a new state-of-the-art on ScanNet test (+0.4 mAP50).
Please see our extended work Mask3D: Mask Transformer for 3D Instance Segmentation accepted at ICRA 2023.
Mix3D: Out-of-Context Data Augmentation for 3D Scenes
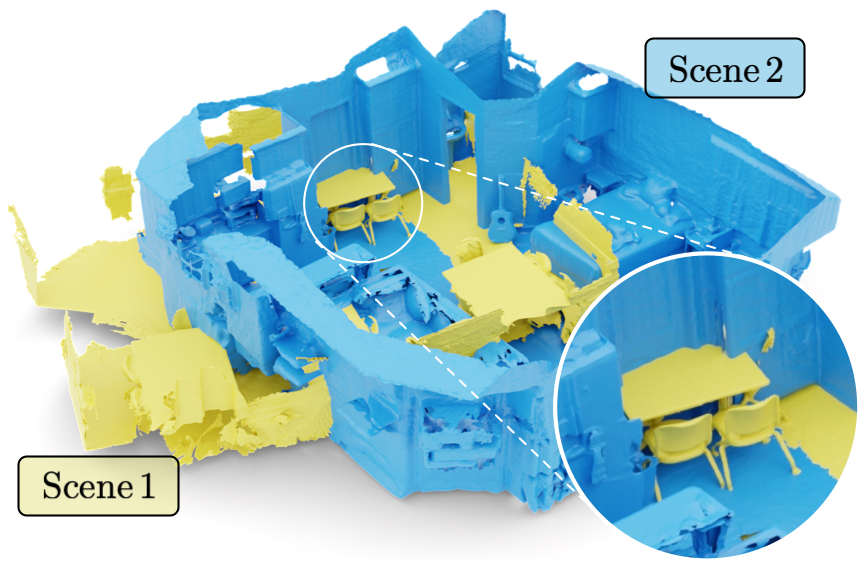
Mix3D is a data augmentation technique for segmenting large-scale 3D scenes. Since scene context helps reasoning about object semantics, current works focus on models with large capacity and receptive fields that can fully capture the global context of an input 3D scene. However, strong contextual priors can have detrimental implications like mistaking a pedestrian crossing the street for a car. In this work, we focus on the importance of balancing global scene context and local geometry, with the goal of generalizing beyond the contextual priors in the training set. In particular, we propose a "mixing" technique which creates new training samples by combining two augmented scenes. By doing so, object instances are implicitly placed into novel out-of-context environments and therefore making it harder for models to rely on scene context alone, and instead infer semantics from local structure as well.
In the paper, we perform detailed analysis to understand the importance of global context, local structures and the effect of mixing scenes. In experiments, we show that models trained with Mix3D profit from a significant performance boost on indoor (ScanNet, S3DIS) and outdoor datasets (SemanticKITTI). Mix3D can be trivially used with any existing method, e.g., trained with Mix3D, MinkowskiNet outperforms all prior state-of-the-art methods by a significant margin on the ScanNet test benchmark 78.1 mIoU.
@inproceedings{Nekrasov213DV,
title = {{Mix3D: Out-of-Context Data Augmentation for 3D Scenes}},
author = {Nekrasov, Alexey and Schult, Jonas and Or, Litany and Leibe, Bastian and Engelmann, Francis},
booktitle = {{International Conference on 3D Vision (3DV)}},
year = {2021}
}
From Points to Multi-Object 3D Reconstruction
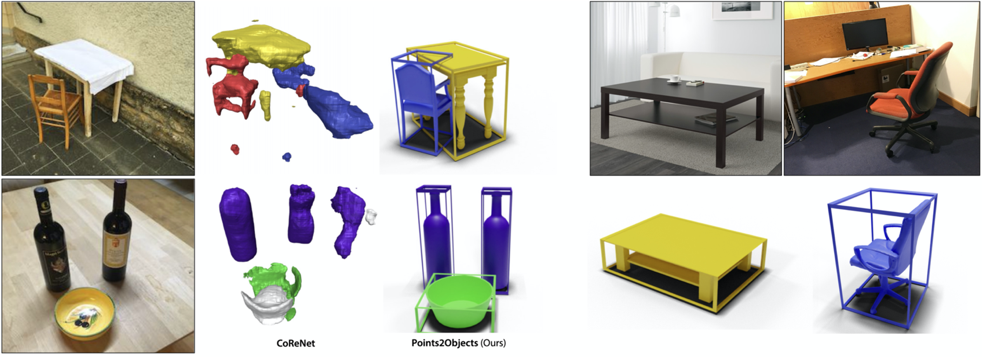
We propose a method to detect and reconstruct multiple 3D objects from a single RGB image. The key idea is to optimize for detection, alignment and shape jointly over all objects in the RGB image, while focusing on realistic and physically plausible reconstructions. To this end, we propose a keypoint detector that localizes objects as center points and directly predicts all object properties, including 9-DoF bounding boxes and 3D shapes -- all in a single forward pass. The proposed method formulates 3D shape reconstruction as a shape selection problem, i.e. it selects among exemplar shapes from a given database. This makes it agnostic to shape representations, which enables a lightweight reconstruction of realistic and visually-pleasing shapes based on CAD-models, while the training objective is formulated around point clouds and voxel representations. A collision-loss promotes non-intersecting objects, further increasing the reconstruction realism. Given the RGB image, the presented approach performs lightweight reconstruction in a single-stage, it is real-time capable, fully differentiable and end-to-end trainable. Our experiments compare multiple approaches for 9-DoF bounding box estimation, evaluate the novel shape-selection mechanism and compare to recent methods in terms of 3D bounding box estimation and 3D shape reconstruction quality.
@inproceedings{Engelmann21CVPR,
title = {{From Points to Multi-Object 3D Reconstruction}},
author = {Engelmann, Francis and Rematas, Konstantinos and Leibe, Bastian and Ferrari, Vittorio},
booktitle = {{IEEE Conference on Computer Vision and Pattern Recognition (CVPR)}},
year = {2021}
}
3D-MPA: Multi Proposal Aggregation for 3D Semantic Instance Segmentation
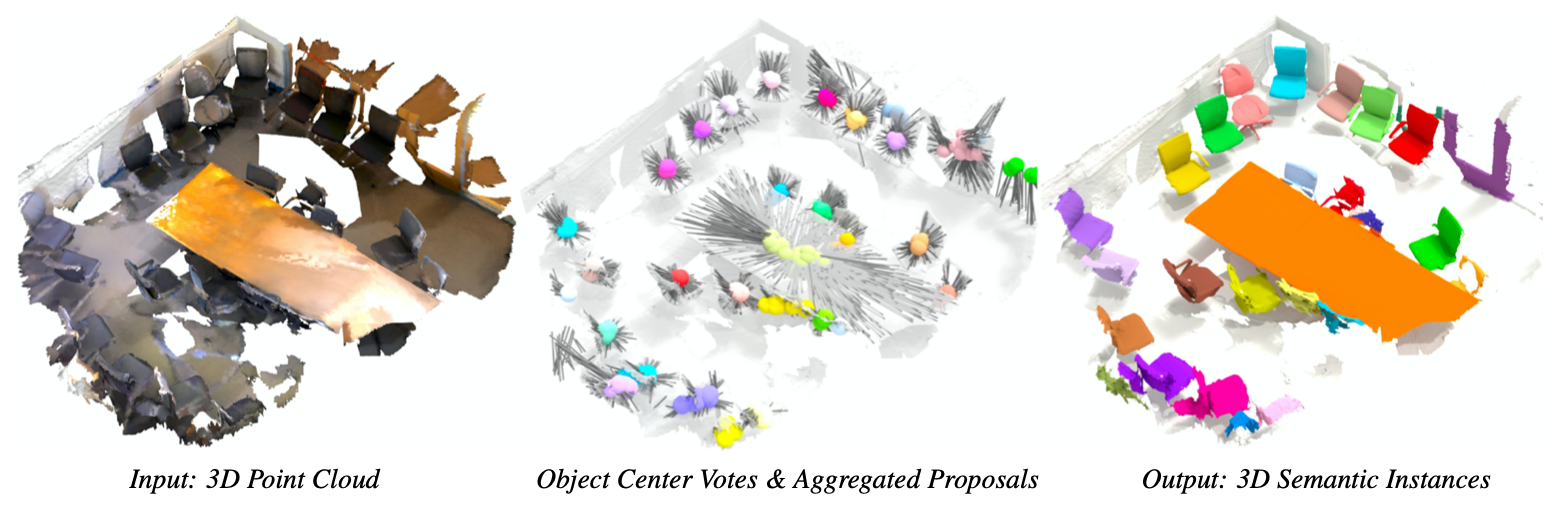
We present 3D-MPA, a method for instance segmentation on 3D point clouds. Given an input point cloud, we propose an object-centric approach where each point votes for its object center. We sample object proposals from the predicted object centers. Then we learn proposal features from grouped point features that voted for the same object center. A graph convolutional network introduces inter-proposal relations, providing higher-level feature learning in addition to the lower-level point features. Each proposal comprises a semantic label, a set of associated points over which we define a foreground-background mask, an objectness score and aggregation features. Previous works usually perform non-maximum-suppression (NMS) over proposals to obtain the final object detections or semantic instances. However, NMS can discard potentially correct predictions. Instead, our approach keeps all proposals and groups them together based on the learned aggregation features. We show that grouping proposals improves over NMS and outperforms previous state-of-the-art methods on the tasks of 3D object detection and semantic instance segmentation on the ScanNetV2 benchmark and the S3DIS dataset.
@inproceedings{Engelmann20CVPR,
title = {{3D-MPA: Multi Proposal Aggregation for 3D Semantic Instance Segmentation}},
author = {Engelmann, Francis and Bokeloh, Martin and Fathi, Alireza and Leibe, Bastian and Nie{\ss}ner, Matthias},
booktitle = {{IEEE Conference on Computer Vision and Pattern Recognition (CVPR)}},
year = {2020}
}
DualConvMesh-Net: Joint Geodesic and Euclidean Convolutions on 3D Meshes
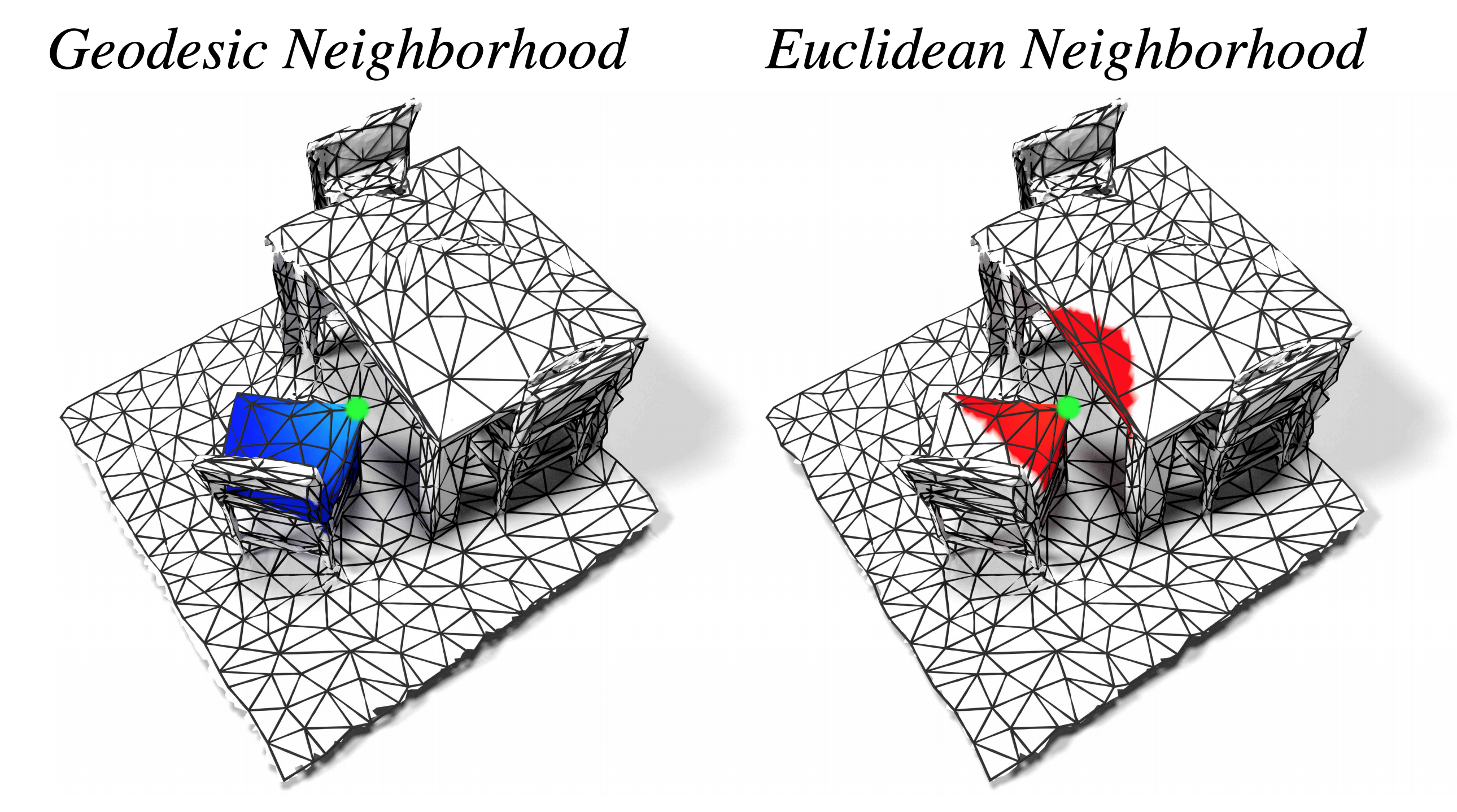
We propose DualConvMesh-Nets (DCM-Net) a family of deep hierarchical convolutional networks over 3D geometric data that combines two types of convolutions. The first type, geodesic convolutions, defines the kernel weights over mesh surfaces or graphs. That is, the convolutional kernel weights are mapped to the local surface of a given mesh. The second type, Euclidean convolutions, is independent of any underlying mesh structure. The convolutional kernel is applied on a neighborhood obtained from a local affinity representation based on the Euclidean distance between 3D points. Intuitively, geodesic convolutions can easily separate objects that are spatially close but have disconnected surfaces, while Euclidean convolutions can represent interactions between nearby objects better, as they are oblivious to object surfaces. To realize a multi-resolution architecture, we borrow well-established mesh simplification methods from the geometry processing domain and adapt them to define mesh-preserving pooling and unpooling operations. We experimentally show that combining both types of convolutions in our architecture leads to significant performance gains for 3D semantic segmentation, and we report competitive results on three scene segmentation benchmarks.
@inproceedings{Schult20CVPR,
author = {Jonas Schult* and
Francis Engelmann* and
Theodora Kontogianni and
Bastian Leibe},
title = {{DualConvMesh-Net: Joint Geodesic and Euclidean Convolutions on 3D Meshes}},
booktitle = {{IEEE Conference on Computer Vision and Pattern Recognition (CVPR)}},
year = {2020}
}
Dilated Point Convolutions: On the Receptive Field Size of Point Convolutions on 3D Point Clouds
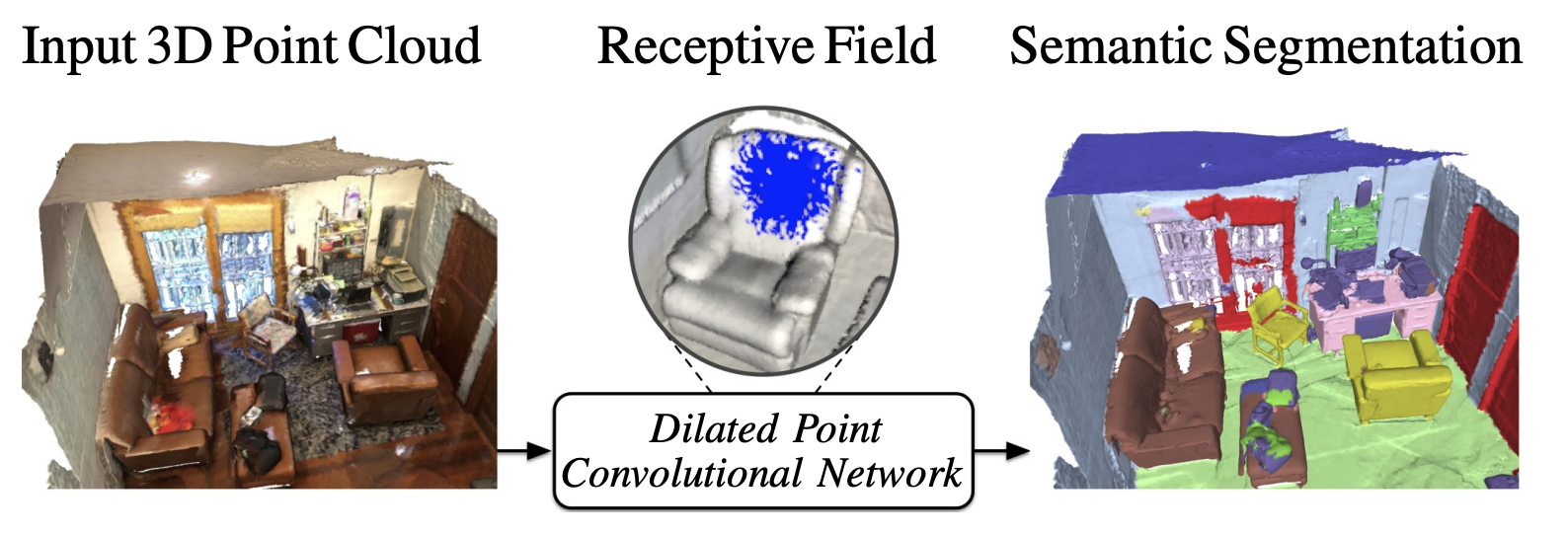
In this work, we propose Dilated Point Convolutions (DPC). In a thorough ablation study, we show that the receptive field size is directly related to the performance of 3D point cloud processing tasks, including semantic segmentation and object classification. Point convolutions are widely used to efficiently process 3D data representations such as point clouds or graphs. However, we observe that the receptive field size of recent point convolutional networks is inherently limited. Our dilated point convolutions alleviate this issue, they significantly increase the receptive field size of point convolutions. Importantly, our dilation mechanism can easily be integrated into most existing point convolutional networks. To evaluate the resulting network architectures, we visualize the receptive field and report competitive scores on popular point cloud benchmarks.
@inproceedings{Engelmann20ICRA,
author = {Engelmann, Francis and Kontogianni, Theodora and Leibe, Bastian},
title = {{Dilated Point Convolutions: On the Receptive Field Size of Point Convolutions on 3D Point Clouds}},
booktitle = {{International Conference on Robotics and Automation (ICRA)}},
year = {2020}
}
3D-BEVIS: Birds-Eye-View Instance Segmentation
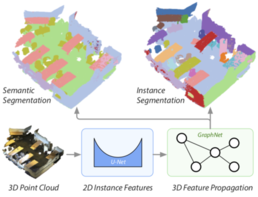
Recent deep learning models achieve impressive results on 3D scene analysis tasks by operating directly on unstructured point clouds. A lot of progress was made in the field of object classification and semantic segmentation. However, the task of instance segmentation is less explored. In this work, we present 3D-BEVIS, a deep learning framework for 3D semantic instance segmentation on point clouds. Following the idea of previous proposal-free instance segmentation approaches, our model learns a feature embedding and groups the obtained feature space into semantic instances. Current point-based methods scale linearly with the number of points by processing local sub-parts of a scene individually. However, to perform instance segmentation by clustering, globally consistent features are required. Therefore, we propose to combine local point geometry with global context information from an intermediate bird's-eye view representation.
@inproceedings{ElichGCPR19,
title = {{3D-BEVIS: Birds-Eye-View Instance Segmentation}},
author = {Elich, Cathrin and Engelmann, Francis and Schult, Jonas and Kontogianni, Theodora and Leibe, Bastian},
booktitle = {{German Conference on Pattern Recognition (GCPR)}},
year = {2019}
}
Know What Your Neighbors Do: 3D Semantic Segmentation of Point Clouds
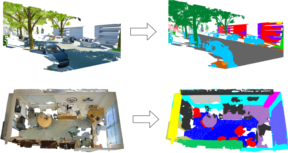
In this paper, we present a deep learning architecture which addresses the problem of 3D semantic segmentation of unstructured point clouds. Compared to previous work, we introduce grouping techniques which define point neighborhoods in the initial world space and the learned feature space. Neighborhoods are important as they allow to compute local or global point features depending on the spatial extend of the neighborhood. Additionally, we incorporate dedicated loss functions to further structure the learned point feature space: the pairwise distance loss and the centroid loss. We show how to apply these mechanisms to the task of 3D semantic segmentation of point clouds and report state-of-the-art performance on indoor and outdoor datasets.
@inproceedings{3dsemseg_ECCVW18,
author = {Francis Engelmann and
Theodora Kontogianni and
Jonas Schult and
Bastian Leibe},
title = {Know What Your Neighbors Do: 3D Semantic Segmentation of Point Clouds},
booktitle = {{IEEE} European Conference on Computer Vision, GMDL Workshop, {ECCV}},
year = {2018}
}
Exploring Spatial Context for 3D Semantic Segmentation of Point Clouds
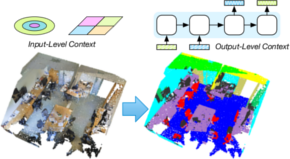
Deep learning approaches have made tremendous progress in the field of semantic segmentation over the past few years. However, most current approaches operate in the 2D image space. Direct semantic segmentation of unstructured 3D point clouds is still an open research problem. The recently proposed PointNet architecture presents an interesting step ahead in that it can operate on unstructured point clouds, achieving decent segmentation results. However, it subdivides the input points into a grid of blocks and processes each such block individually. In this paper, we investigate the question how such an architecture can be extended to incorporate larger-scale spatial context. We build upon PointNet and propose two extensions that enlarge the receptive field over the 3D scene. We evaluate the proposed strategies on challenging indoor and outdoor datasets and show improved results in both scenarios.
» Show BibTeX
@inproceedings{3dsemseg_ICCVW17,
author = {Francis Engelmann and
Theodora Kontogianni and
Alexander Hermans and
Bastian Leibe},
title = {Exploring Spatial Context for 3D Semantic Segmentation of Point Clouds},
booktitle = {{IEEE} International Conference on Computer Vision, 3DRMS Workshop, {ICCV}},
year = {2017}
}
Keyframe-Based Visual-Inertial Online SLAM with Relocalization
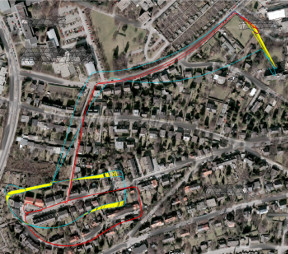
Complementing images with inertial measurements has become one of the most popular approaches to achieve highly accurate and robust real-time camera pose tracking. In this paper, we present a keyframe-based approach to visual-inertial simultaneous localization and mapping (SLAM) for monocular and stereo cameras. Our method is based on a real-time capable visual-inertial odometry method that provides locally consistent trajectory and map estimates. We achieve global consistency in the estimate through online loop-closing and non-linear optimization. Furthermore, our approach supports relocalization in a map that has been previously obtained and allows for continued SLAM operation. We evaluate our approach in terms of accuracy, relocalization capability and run-time efficiency on public benchmark datasets and on newly recorded sequences. We demonstrate state-of-the-art performance of our approach towards a visual-inertial odometry method in recovering the trajectory of the camera.
@article{Kasyanov2017_VISLAM,
title={{Keyframe-Based Visual-Inertial Online SLAM with Relocalization}},
author={Anton Kasyanov and Francis Engelmann and J\"org St\"uckler and Bastian Leibe},
booktitle={{IEEE/RSJ} International Conference on Intelligent Robots and Systems {(IROS)}},
year={2017}
}
SAMP: Shape and Motion Priors for 4D Vehicle Reconstruction
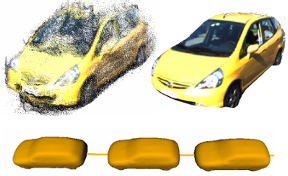
Inferring the pose and shape of vehicles in 3D from a movable platform still remains a challenging task due to the projective sensing principle of cameras, difficult surface properties, e.g. reflections or transparency, and illumination changes between images. In this paper, we propose to use 3D shape and motion priors to regularize the estimation of the trajectory and the shape of vehicles in sequences of stereo images. We represent shapes by 3D signed distance functions and embed them in a low-dimensional manifold. Our optimization method allows for imposing a common shape across all image observations along an object track. We employ a motion model to regularize the trajectory to plausible object motions. We evaluate our method on the KITTI dataset and show state-of-the-art results in terms of shape reconstruction and pose estimation accuracy.
@inproceedings{EngelmannWACV17_samp,
author = {Francis Engelmann and J{\"{o}}rg St{\"{u}}ckler and Bastian Leibe},
title = {{SAMP:} Shape and Motion Priors for 4D Vehicle Reconstruction},
booktitle = {{IEEE} Winter Conference on Applications of Computer Vision,
{WACV}},
year = {2017}
}
Joint Object Pose Estimation and Shape Reconstruction in Urban Street Scenes Using 3D Shape Priors
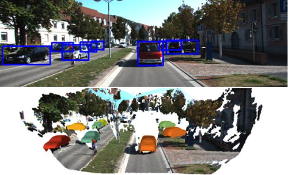
Estimating the pose and 3D shape of a large variety of instances within an object class from stereo images is a challenging problem, especially in realistic conditions such as urban street scenes. We propose a novel approach for using compact shape manifolds of the shape within an object class for object segmentation, pose and shape estimation. Our method first detects objects and estimates their pose coarsely in the stereo images using a state-of-the-art 3D object detection method. An energy minimization method then aligns shape and pose concurrently with the stereo reconstruction of the object. In experiments, we evaluate our approach for detection, pose and shape estimation of cars in real stereo images of urban street scenes. We demonstrate that our shape manifold alignment method yields improved results over the initial stereo reconstruction and object detection method in depth and pose accuracy.
» Show BibTeX
@inproceedings{EngelmannGCPR16_shapepriors,
title = {Joint Object Pose Estimation and Shape Reconstruction in Urban Street Scenes Using {3D} Shape Priors},
author = {Francis Engelmann and J\"org St\"uckler and Bastian Leibe},
booktitle = {Proc. of the German Conference on Pattern Recognition (GCPR)},
year = {2016}}
Multi-Scale Object Candidates for Generic Object Tracking in Street Scenes
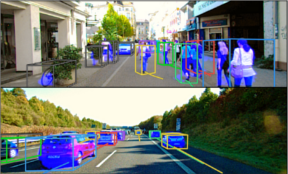
Most vision based systems for object tracking in urban environments focus on a limited number of important object categories such as cars or pedestrians, for which powerful detectors are available. However, practical driving scenarios contain many additional objects of interest, for which suitable detectors either do not yet exist or would be cumbersome to obtain. In this paper we propose a more general tracking approach which does not follow the often used tracking-by- detection principle. Instead, we investigate how far we can get by tracking unknown, generic objects in challenging street scenes. As such, we do not restrict ourselves to only tracking the most common categories, but are able to handle a large variety of static and moving objects. We evaluate our approach on the KITTI dataset and show competitive results for the annotated classes, even though we are not restricted to them.
@inproceedings{Osep16ICRA,
title={Multi-Scale Object Candidates for Generic Object Tracking in Street
Scenes},
author={O\v{s}ep, Aljo\v{s}a and Hermans, Alexander and Engelmann, Francis and Klostermann, Dirk and and Mathias, Markus and Leibe, Bastian},
booktitle={ICRA},
year={2016}
}
Multiple Target Tracking for Marker-less Augmented Reality
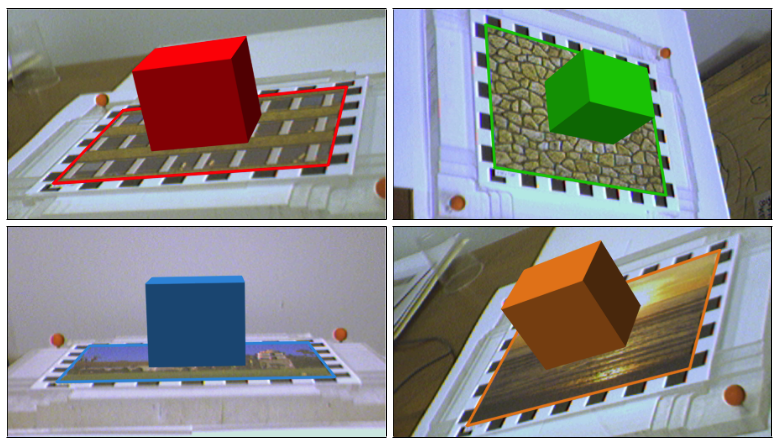
In this work, we implemented an AR framework for planar targets based on the ORB feature-point descriptor. The main components of the framework are a detector, a tracker and a graphical overlay. The detector returns a homography that maps the model- image onto the target in the camera-image. The homography is estimated from a set of feature-point correspondences using the Direct Linear Transform (DLT) algorithm and Levenberg-Marquardt (LM) optimization. The outliers in the set of feature-point correspondences are removed using RANSAC. The tracker is based on the Kalman filter, which applies a consistent dynamic movement on the target. In a hierarchical matching scheme, we extract additional matches from consecutive frames and perspectively transformed model-images, which yields more accurate and jitter-free homography estimations. The graphical overlay computes the six-degree-of-freedom (6DoF) pose from the estimated homography. Finally, to visualize the computed pose, we draw a cube on the surface of the tracked target. In the evaluation part, we analyze the performance of our system by looking at the accuracy of the estimated homography and the ratio of correctly tracked frames. The evaluation is based on the ground truth provided by two datasets. We evaluate most components of the framework under different target movements and lighting conditions. In particular, we proof that our framework is robust against considerable perspective distortion and show the benefit of using the hierarchical matching scheme to minimize jitter and improve accuracy.