Profile
![]() |
Dr. Stefan Breuers |
Teaching
Computer Vision, WS 13/14
Computer Vision 2, SS 14
Computer Vision, WS 15/16
Computer Vision, WS 16/17
Seminar SS 14, SS 15, SS 16, WS 16/17, SS 18
Proseminar WS 18/19
Students
Shishan Yang (Hiwi, Master thesis)
Killian Halloum (Bachelor thesis)
Kersten Schuster (Hiwi)
Antonia Breuer (Hiwi, Master thesis)
Judith Hermanns (Hiwi, Master thesis)
Reviews
CVPR 2014 (1 paper)
GCPR 2014 (2 paper)
TOR 2014 (1 paper)
GCPR 2015 (1 paper)
IROS 2015 (1 paper)
ECCV 2016 (5 paper)
IROS 2016 (2 paper)
CVPR 2017 (5 paper) Outstanding Reviewer Award
CVPR 2018 (2 paper)
ACCV 2018 (2 paper)
Publications
Large-Scale Object Mining for Object Discovery from Unlabeled Video
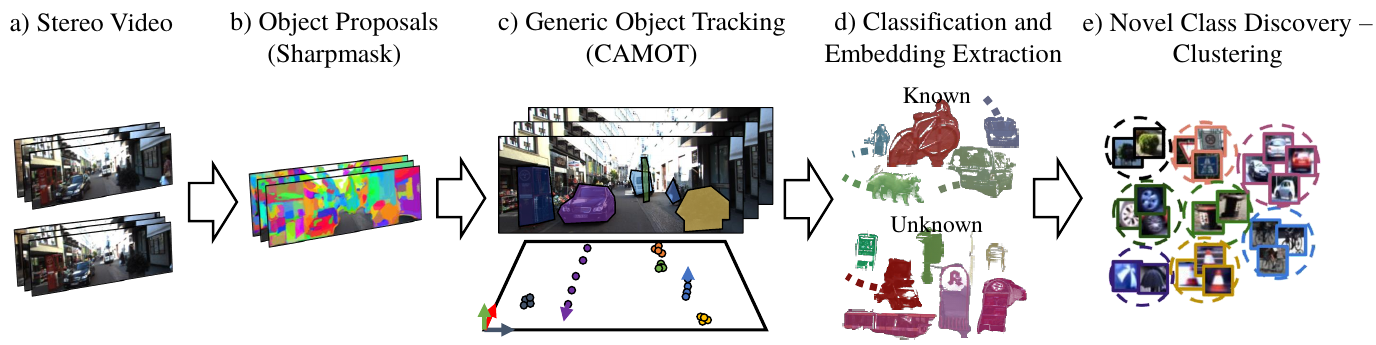
This paper addresses the problem of object discovery from unlabeled driving videos captured in a realistic automotive setting. Identifying recurring object categories in such raw video streams is a very challenging problem. Not only do object candidates first have to be localized in the input images, but many interesting object categories occur relatively infrequently. Object discovery will therefore have to deal with the difficulties of operating in the long tail of the object distribution. We demonstrate the feasibility of performing fully automatic object discovery in such a setting by mining object tracks using a generic object tracker. In order to facilitate further research in object discovery, we will release a collection of more than 360'000 automatically mined object tracks from 10+ hours of video data (560'000 frames). We use this dataset to evaluate the suitability of different feature representations and clustering strategies for object discovery.
@article{Osep19ICRA,
author = {O\v{s}ep, Aljo\v{s}a and Voigtlaender, Paul and Luiten, Jonathon and Breuers, Stefan and Leibe, Bastian},
title = {Large-Scale Object Mining for Object Discovery from Unlabeled Video},
journal = {ICRA},
year = {2019}
}
Towards Large-Scale Video Video Object Mining
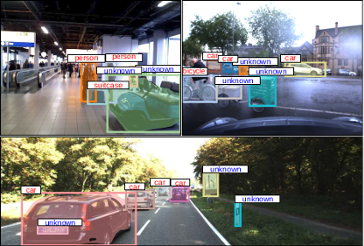
We propose to leverage a generic object tracker in order to perform object mining in large-scale unlabeled videos, captured in a realistic automotive setting. We present a dataset of more than 360'000 automatically mined object tracks from 10+ hours of video data (560'000 frames) and propose a method for automated novel category discovery and detector learning. In addition, we show preliminary results on using the mined tracks for object detector adaptation.
@article{OsepVoigtlaender18ECCVW,
title={Towards Large-Scale Video Object Mining},
author={Aljo\v{s}a O\v{s}ep and Paul Voigtlaender and Jonathon Luiten and Stefan Breuers and Bastian Leibe},
journal={ECCV 2018 Workshop on Interactive and Adaptive Learning in an Open World},
year={2018}
}
Detection-Tracking for Efficient Person Analysis: The DetTA Pipeline
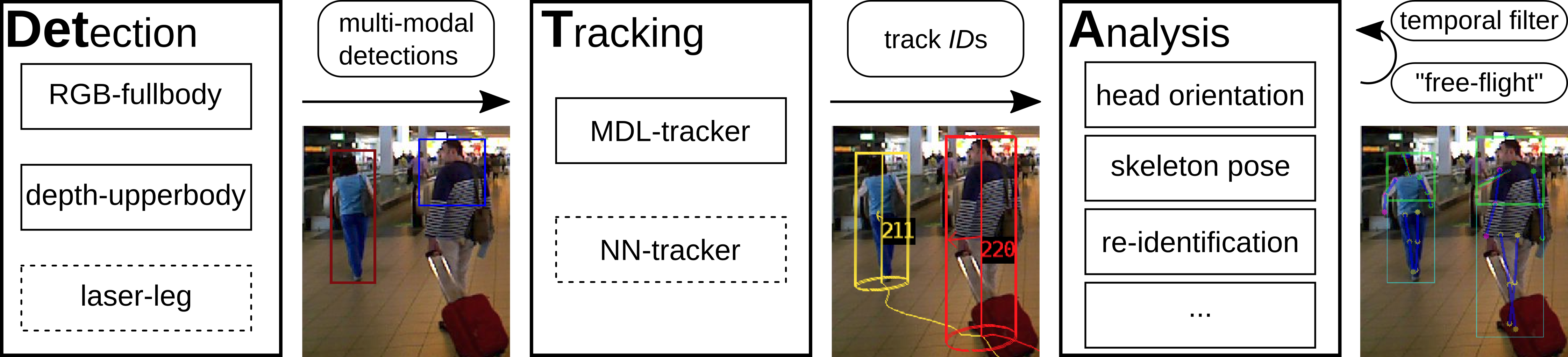
TL;DR: Detection+Tracking+{head orientation,skeleton} analysis. Smooth per-track enables filtering outliers as well as a "free flight" mode where expensive analysis modules are run with a stride, dramatically increasing runtime performance at almost no loss of prediction quality.
In the past decade many robots were deployed in the wild, and people detection and tracking is an important component of such deployments. On top of that, one often needs to run modules which analyze persons and extract higher level attributes such as age and gender, or dynamic information like gaze and pose. The latter ones are especially necessary for building a reactive, social robot-person interaction.
In this paper, we combine those components in a fully modular detection-tracking-analysis pipeline, called DetTA. We investigate the benefits of such an integration on the example of head and skeleton pose, by using the consistent track ID for a temporal filtering of the analysis modules’ observations, showing a slight improvement in a challenging real-world scenario. We also study the potential of a so-called “free-flight” mode, where the analysis of a person attribute only relies on the filter’s predictions for certain frames. Here, our study shows that this boosts the runtime dramatically, while the prediction quality remains stable. This insight is especially important for reducing power consumption and sharing precious (GPU-)memory when running many analysis components on a mobile platform, especially so in the era of expensive deep learning methods.
@article{BreuersBeyer2018Arxiv,
title = {{Detection-Tracking for Efficient Person Analysis: The DetTA Pipeline}},
author = {Breuers*, Stefan and Beyer*, Lucas and Rafi, Umer and Leibe, Bastian},
journal = {arXiv preprint arXiv:TBD},
year = {2018}
}
Large-Scale Object Discovery and Detector Adaptation from Unlabeled Video
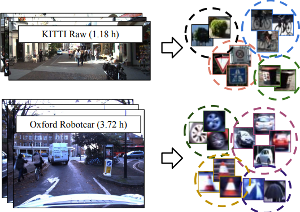
We explore object discovery and detector adaptation based on unlabeled video sequences captured from a mobile platform. We propose a fully automatic approach for object mining from video which builds upon a generic object tracking approach. By applying this method to three large video datasets from autonomous driving and mobile robotics scenarios, we demonstrate its robustness and generality. Based on the object mining results, we propose a novel approach for unsupervised object discovery by appearance-based clustering. We show that this approach successfully discovers interesting objects relevant to driving scenarios. In addition, we perform self-supervised detector adaptation in order to improve detection performance on the KITTI dataset for existing categories. Our approach has direct relevance for enabling large-scale object learning for autonomous driving.
@article{OsepVoigtlaender18arxiv,
title={Large-Scale Object Discovery and Detector Adaptation from Unlabeled Video},
author={Aljo\v{s}a O\v{s}ep and Paul Voigtlaender and Jonathon Luiten and Stefan Breuers and Bastian Leibe},
journal={arXiv preprint arXiv:1712.08832},
year={2018}
}
Towards a Principled Integration of Multi-Camera Re-Identification and Tracking through Optimal Bayes Filters
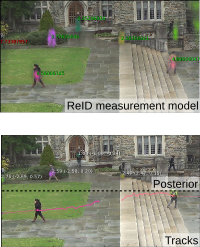
TL;DR: Explorative paper. Learn a Triplet-ReID net, embed the full image. Keep embeddings of known tracks, correlate them with image embeddings and use that as measurement model in a Bayesian filtering tracker. MOT score is mediocre, but framework is theoretically pleasing.
With the rise of end-to-end learning through deep learning, person detectors and re-identification (ReID) models have recently become very strong. Multi-camera multi-target (MCMT) tracking has not fully gone through this transformation yet. We intend to take another step in this direction by presenting a theoretically principled way of integrating ReID with tracking formulated as an optimal Bayes filter. This conveniently side-steps the need for data-association and opens up a direct path from full images to the core of the tracker. While the results are still sub-par, we believe that this new, tight integration opens many interesting research opportunities and leads the way towards full end-to-end tracking from raw pixels.
@article{BeyerBreuers2017Arxiv,
author = {Lucas Beyer and
Stefan Breuers and
Vitaly Kurin and
Bastian Leibe},
title = {{Towards a Principled Integration of Multi-Camera Re-Identification
and Tracking through Optimal Bayes Filters}},
journal = {{2017 IEEE Conference on Computer Vision and Pattern Recognition Workshops (CVPRW)}},
year = {2017},
pages ={1444-1453},
}
On Multi-Modal People Tracking from Mobile Platforms in Very Crowded and Dynamic Environments
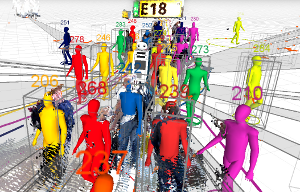
Tracking people is a key technology for robots and intelligent systems in human environments. Many person detectors, filtering methods and data association algorithms for people tracking have been proposed in the past 15+ years in both the robotics and computer vision communities, achieving decent tracking performances from static and mobile platforms in real-world scenarios. However, little effort has been made to compare these methods, analyze their performance using different sensory modalities and study their impact on different performance metrics. In this paper, we propose a fully integrated real-time multi-modal laser/RGB-D people tracking framework for moving platforms in environments like a busy airport terminal. We conduct experiments on two challenging new datasets collected from a first-person perspective, one of them containing very dense crowds of people with up to 30 individuals within close range at the same time. We consider four different, recently proposed tracking methods and study their impact on seven different performance metrics, in both single and multi-modal settings. We extensively discuss our findings, which indicate that more complex data association methods may not always be the better choice, and derive possible future research directions.
» Show BibTeX
@incollection{linder16multi,
title={On Multi-Modal People Tracking from Mobile Platforms in Very Crowded and Dynamic Environments},
author={Linder, Timm and Breuers, Stefan and Leibe, Bastian and Arras, Kai Oliver},
booktitle={ICRA},
year={2016},
}
Exploring Bounding Box Context for Multi-Object Tracker Fusion
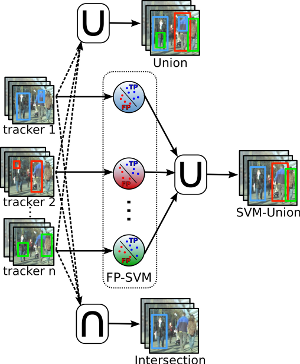
Many multi-object-tracking (MOT) techniques have been developed over the past years. The most successful ones are based on the classical tracking-by-detection approach. The different methods rely on different kinds of data association, use motion and appearance models, or add optimization terms for occlusion and exclusion. Still, errors occur for all those methods and a consistent evaluation has just started. In this paper we analyze three current state-of-the-art MOT trackers and show that there is still room for improvement. To that end, we train a classifier on the trackers' output bounding boxes in order to prune false positives. Furthermore, the different approaches have different strengths resulting in a reduced false negative rate when combined. We perform an extensive evaluation over ten common evaluation sequences and consistently show improved performances by exploiting the strengths and reducing the weaknesses of current methods.
@inproceedings{breuersWACV16,
title={Exploring Bounding Box Context for Multi-Object Tracker Fusion},
author={Breuers, Stefan and Yang, Shishan and Mathias, Markus and Leibe, Bastian},
booktitle={WACV},
year={2016}
}
SPENCER: A Socially Aware Service Robot for Passenger Guidance and Help in Busy Airports
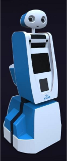
We present an ample description of a socially compliant mobile robotic platform, which is developed in the EU-funded project SPENCER. The purpose of this robot is to assist, inform and guide passengers in large and busy airports. One particular aim is to bring travellers of connecting flights conveniently and efficiently from their arrival gate to the passport control. The uniqueness of the project stems from the strong demand of service robots for this application with a large potential impact for the aviation industry on one side, and on the other side from the scientific advancements in social robotics, brought forward and achieved in SPENCER. The main contributions of SPENCER are novel methods to perceive, learn, and model human social behavior and to use this knowledge to plan appropriate actions in real- time for mobile platforms. In this paper, we describe how the project advances the fields of detection and tracking of individuals and groups, recognition of human social relations and activities, normative human behavior learning, socially-aware task and motion planning, learning socially annotated maps, and conducting empir- ical experiments to assess socio-psychological effects of normative robot behaviors.
@article{triebel2015spencer,
title={SPENCER: a socially aware service robot for passenger guidance and help in busy airports},
author={Triebel, Rudolph and Arras, Kai and Alami, Rachid and Beyer, Lucas and Breuers, Stefan and Chatila, Raja and Chetouani, Mohamed and Cremers, Daniel and Evers, Vanessa and Fiore, Michelangelo and Hung, Hayley and Islas Ramírez, Omar A. and Joosse, Michiel and Khambhaita, Harmish and Kucner, Tomasz and Leibe, Bastian and Lilienthal, Achim J. and Linder, Timm and Lohse, Manja and Magnusson, Martin and Okal, Billy and Palmieri, Luigi and Rafi, Umer and Rooij, Marieke van and Zhang, Lu},
journal={Field and Service Robotics (FSR)
year={2015},
publisher={University of Toronto}
}
Improved Ramsey-based Büchi Complementation
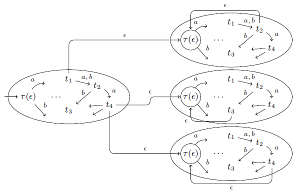
We consider complementing Büchi automata by applying the Ramsey-based approach, which is the original approach already used by Büchi and later improved by Sistla et al. We present several heuristics to reduce the state space of the resulting complement automaton and provide experimental data that shows that our improved construction can compete (in terms of finished complementation tasks) also in practice with alternative constructions like rank-based complementation. Furthermore, we show how our techniques can be used to improve the Ramsey-based complementation such that the asymptotic upper bound for the resulting complement automaton is 2^O(n log n) instead of 2^O(n2).
@incollection{breuers2012improved,
title={Improved Ramsey-based B{\"u}chi Complementation},
author={Breuers, Stefan and L{\"o}ding, Christof and Olschewski, J{\"o}rg},
booktitle={Foundations of Software Science and Computational Structures},
pages={150--164},
year={2012},
publisher={Springer}
}